Download English (US), New Release 410.130. CUDA driver update to support CUDA Toolkit 10.0 and macOS 10.13.6. NVIDIA® CUDA Toolkit 11.0 no longer supports development or running applications on macOS. While there are no tools which use macOS as a target environment, NVIDIA is making macOS host versions of these tools that you can launch profiling and debugging sessions on supported target platforms. Download the GeForce NOW Cloud Gaming Service. Most Window PCs, Mac Computers, and Android Devices are Supported. Nvidia GPUs receive driver updates soon after each version update of OS X. Only one driver is released by Nvidia and it includes support for all of their modern GPUs. You will not find individually named Nvidia drivers for OS X, they are all titled 'Quadro & Geforce Mac OS X Driver Release xxx.xx.xxxxx'.
NVIDIA® CUDA Toolkit 11.1 Update 1 no longer supports development or running applications on macOS. While there are no tools which use macOS as a target environment, NVIDIA is making macOS host versions of these tools that you can launch profiling and debugging sessions on supported target platforms.
You may download all these tools here. Note that the Nsight tools provide the ability to download these macOS host versions on their respective product pages.
Please visit each tool's overview page for more information about the tool and its supported target platforms.
The macOS host tools provided are:
- Nsight Systems - a system profiler and timeline trace tool supporting Pascal and newer GPUs
- Nsight Compute - a CUDA kernel profiler supporting Volta and new GPUs
- Visual Profiler - a CUDA kernel and system profiler and timeline trace tool supporting older GPUs (see installation instructions, below)
- cuda-gdb - a GPU and CPU CUDA application debugger (see installation instructions, below)
Revision History
Instructions for installing cuda-gdb on the macOS
- This tar archive holds the distribution of the CUDA 11.1 Update 1 cuda-gdb cuda-gdb debugger front-end for macOS.
Native macOS debugging is not supported in this release. Remote debugging from a macOS host to other CUDA enabled targets, however, is supported.
Supported Mac platforms: macOS 10.13
- To install:
- Create an installation directory
INSTALL_DIR=$HOME/cuda-gdb-darwin-11.1
mkdir $INSTALL_DIR
cd $INSTALL_DIR
- Download the cuda-gdb-darwin-11.1.105.tar.gz tar archive into
$INSTALL_DIR
above - Unpack the tar archive
tar fxvz cuda-gdb-darwin-11.1.105.tar.gz
- Add the bin directory to your path
PATH=$INSTALL_DIR/bin:$PATH
- Run cuda-gdb --version to confirm you're picking up the correct binaries
cuda-gdb --version
- Download version: 8u144-b01 (Zulu: 8.23.0.3) .dmg.zip.tar.gz
- Download version: Zulu 8.23.0.3 (build 1.8.0_144-b01 .zip
- Hardware acceleration for most popular video framework
- Leverages FFmpeg’s Audio codec, stream muxing, and RTP protocols
- Available for Windows, Linux
- You can now use FFMPEG to accelerate video encoding and decoding using NVENC and NVDEC, respectively.
- AV1 Main profile decoding up to level 6.0x
- Decoding Histogram collection for AV1, HEVC, H264 and VP9
- Alpha Layer Encoding in HEVC
- Temporal SVC Encoding in H.264
- 'Recommended NVENC Settings' in NVECODE API Programming Guide, included in the Video Codec SDK
- 'Writing an Efficient Decode Application' in NVDECODE API Programming Guide, included in the Video Codec SDK
- Download older legacy versions of NVENC SDK and Video Codec SDK
- DownloadCUDA Toolkit
- DownloadFFmpeg
- Download Video Test Sources (YUV RAW 1080p Files - Heavy Hand video input)
- Blog - VPF: Hardware-Accelerated Video Processing Framework in Python
- Blog - Turing H.264 Video Encoding Speed and Quality
- Blog - New GeForce-Optimized OBS and RTX Encoder Enables Pro-Quality Broadcasting on a Single PC
- Partner ProductStreamline live streaming system reference design
You should see the following output:
Discover the innovative world of Apple and shop everything iPhone, iPad, Apple Watch, Mac, and Apple TV, plus explore accessories, entertainment, and expert device support. Get instant credit at the Apple Store. Trade in your eligible device at the Apple Store for instant credit toward your next purchase, or get an Apple Store Gift Card you can use anytime. If your device isn‘t eligible for credit, we‘ll recycle it for free. You must be at least 18 years old to be eligible to trade in for credit or for an Apple Store Gift Card. Not all devices are eligible for credit. More details are available from Apple’s Mac trade‑in partner and Apple’s iPhone, iPad, and Apple Watch trade‑in partner for trade-in and recycling of eligible devices. Apple reserves the right to refuse or limit the quantity of any device for any reason. In the Apple Store: Offer only available on presentation of a valid photo ID. Value of your current device may be applied toward purchase of a new Apple device. Offer may not be available in all stores. Some stores may have additional requirements. Tips to avoid becoming the victim of a scam. If you are NOT purchasing an item from Apple — such as from the Apple Store, iTunes Store, App Store, Apple Books, or for an Apple Music subscription or iCloud storage — do NOT make a payment with an Apple Gift Card, App Store & iTunes Gift Card, or Apple Store. Apple gift card for mac store.
- NVIDIA (R) CUDA Debugger
11.1 release
Portions Copyright (C) 2007-2020 NVIDIA Corporation
GNU gdb (GDB) 8.3.1
Copyright (C) 2019 Free Software Foundation, Inc.
License GPLv3+: GNU GPL version 3 or later
for more information:
- https://docs.nvidia.com/cuda/profiler-users-guide/index.html#visual
Notes about JRE Requirements when using Visual Profiler on the macOS
- OpenJDK provides an open-source (and standards compliant) implementation of a Java compliant JVM.
Binaries are provided by various vendors such as Oracle, Azul Systems (Zulu), Amazon, Red Hat, IBM, etc.
- Visual Profiler needs to use an older version of Java, specifically JRE update 151, to work correctly.
This is currently not offered by Oracle JDK but is provided by Azul Systems (Zulu).
- The Bazel Build project also uses the Zulu builds of OpenJDK.
- Download JDK 8.0.144 to get JRE update 151:
Nvidia Quadro K5000 For Mac Download
Revision History

Current Version
Version Archive
If you are looking to make use of the dedicated decoding/encoding hardware on your GPU in an existing application you can leverage the integration already available in FFmpeg. FFmpeg should be used for evaluation or quick integration, but it may not provide control over every encoder parameter. NVDECODE and NVENCODE APIs should be used for low-level granular control over various encode/decode parameters and if you want to directly tap into the hardware decoder/encoder. This access is available through the Video Codec SDK.
Cross-platform solution to record, convert, and stream audio and video. Includes NVIDIA Video Hardware Acceleration
10/14/2020
DOWNLOAD NOW Source Code
What's new in Video Codec SDK 11
System Requirements for Video Codec SDK 11

Architecture | x64 and ppc64le |
Operating System | Windows 7, 8, 10, Server 2008 R2, Server 2012, and Linux |
Dependencies | NVENCODE API - NVIDIA Quadro, Tesla, GRID or GeForce products with Kepler, Maxwell, Pascal and Turing generation GPUs. NVDECODE API - NVIDIA Quadro, Tesla, GRID or GeForce products with Fermi, Kepler, Maxwell, Pascal and Turing generation GPUs. GPU Support Matrix NVIDIA Windows display driver 456.71 or newer NVIDIA Linux display driver 455.28 or newer DirectX SDK (Windows only) CUDA 11.0 Toolkit |
Development Environment | Windows: Visual Studio 2013/2015/2017/2019 Linux: gcc 4.8 or higher |
Documentation and Samples
Online Documentation
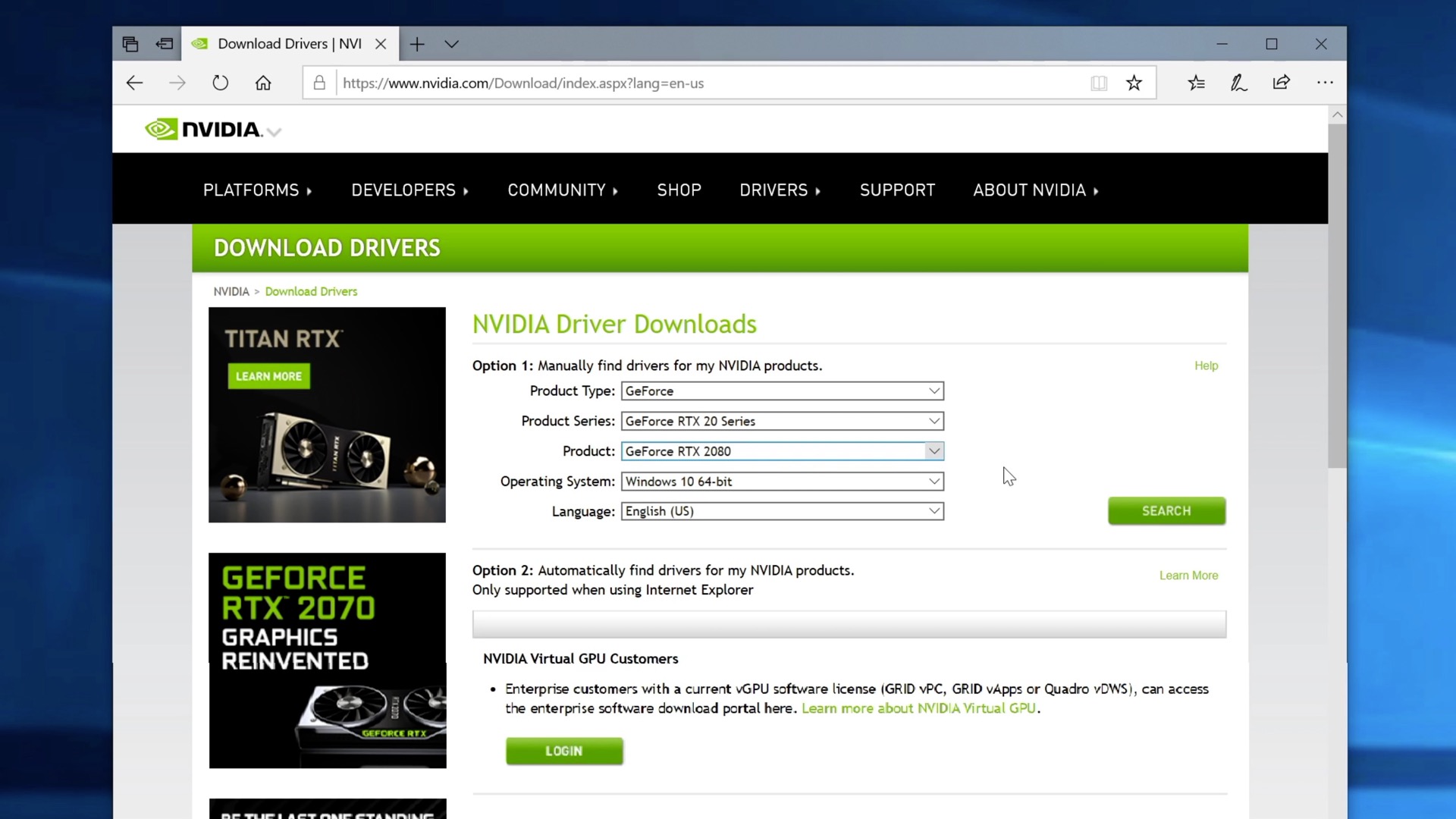
For convenience, NVDECODE API documentation and sample applications are also included in the CUDA Toolkit, in addition to the Video Codec SDK download package.
Note: For Video Codec SDK 7.0 and later, NVCUVID has been renamed to NVDECODE API.
FAQ
Checkpoint vpn client for mac catalina. A: After you download the SDK, please refer to the 'ReadMe.txt' which lists the minimum required display driver version. You need to install the right drivers or else the SDK will fail to start and this is the first thing you should check in case there is an initialization failure.
A: The support matrix is listed https://developer.nvidia.com/video-encode-decode-gpu-support-matrix. The client application should also query the capabilities using the respective capability APIs before enabling any feature.
A: We strongly recommend all application developers to go through the programming guides in detail before writing any application. In particular, for some hints on this question, please go through the following sections in the documentation. These sections provide valuable tips for optimizing latency/memory utilization and choosing the right settings for different use-cases.
A: Video encoding latency consists of two components: (a) Latency due to encoding algorithm (e.g. B-frames, look-ahead, VBV buffering), and (b) Latency due to the processing required to encode the bits using hardware or software. For a typical end-to-end streaming scenario to incur low latency, it is important to lower both components as much as possible. Typically, latency in (a) can be minimized by choosing infinite GOP with IPPPP.. structure, no look-ahead and lowest possible VBV buffer for the given bitrate and available channel bandwidth, without giving away too much of encoding quality. Each of these can all be set via NVENCODE API. In Video SDK 10.0 and above, setting the tuning info to low-latency or ultra-low-latency will set most of these parameters automatically. Latency contributed by (b) can be minimized by choosing the correct preset, and rate control mode with correct number of rate control passes. Naturally, 2-pass requires more time to encode than 1-pass rate control mode. Running quarter-resolution first pass requires less time than running both passes at full resolution.
In addition to the above, the overall encoding latency is also affected by efficient application design (or lack thereof). Since NVENC can run in parallel to CUDA and graphics workload, it is important to ensure that the NVENC pipeline is kept fed with data and the context switches between NVENC pre-processing (which uses small amount of CUDA bandwidth) and other graphics/CUDA workload are minimized. The specifics of this depend on the workload, but should be analyzed using a tool such as GPUView (available as a part of Windows Performance Toolkit).
A: The Video Codec SDK provides samples specifically designed to give optimal performance. Please refer to applications with suffix '…perf' inside the Video Codec SDK. User can run these applications for measuring maximum throughput. The samples in the Optical Flow SDK are optimized for performance.
A: First of all, it is important to note that the aggregate video encoding performance of GPUs is not solely defined by the raw number of NVENCs on the GPU silicon. As anyone familiar with video encoding will know, talking about video encoding performance without any reference to encoding quality is meaningless. For example, one can encode a video at blazing fast speed, without any regard to quality and claim extremely high performance, doubling the performance on GPUs with multiple NVENC engines. But such usage may not be of much use in practical situations. Therefore, it is important to think of encoding performance at a specific quality. NVIDIA encoding benchmarks use the bitrate savings compared with open source encoders x264 and x265's medium preset output, as a measure of the encoding quality. The performance vs. quality spectrum thus obtained is published for various generations of GPUs on Video Codec SDK web site. Most of the commonly used presets on Pascal have an equivalent preset in Turing with similar quality and 2x performance, thereby making it possible to get the same performance from both GPU generations, despite Turing GPUs having only 1 NVENC engine. This requires the application to choose appropriate encoding settings, depending upon the GPU in use. For low-latency presets and tuning, Turing NVENC provides equivalent settings to achieve higher performance per NVENC than Pascal NVENC for latency tolerant encoding. For latency-sensitive (low-latency) encoding, Turing NVENC does not provide 2x performance, but that's not needed because most of the low-latency scenarios are bottlenecked by the graphics/CUDA utilization and not NVENC utilization.
Mac os x apps free download. There are many seemingly small additions and changes that make bottom-line improvements to your overall computing, including a quick way to respond directly from notifications.
In short, despite the reduction of number of NVENCs from Pascal to Turing, one should be able to achieve equivalent encoding performance per GPU, in most practical use cases by adjusting the encoding settings to normalize the encoding quality.
A: NVENCODE API expose APIs which allow users to query the maximum API versions supported by the underlying driver. Depending on the maximum API version supported by driver, the application can launch code at runtime compiled with the appropriate API.
A: For decoder, please refer to the NVDEC application note included in the SDK documentation to get an idea about performance. For encoder, the answer depends on many factors, some of which include: GPU in use and its clock speed, settings used for encoding (i.e. encode quality), memory bandwidth available, application design. It is especially important to note that GPU encoding performance is always tied to the encoding quality, and the performance can vary greatly depending upon the chosen settings. For example, B-frames, 2-pass rate control mode, or look-ahead will improve the encoding quality at the cost of performance. Encoding presets also influence quality vs performance trade-off significantly. Please refer to the table containing indicative performance figures for the video encoder in NVENC application note included in the SDK package.
A: Create separate Cuda streams for encode and decode. For NVDECODEAPI and NVENCODEAPI you can specify the stream where you want to Cuda kernels using CUVIDPROCPARAMS::output_stream and NvEncSetIOCudaStreams(.) respectively.
Support
Our forum community is where Developers can ask questions, share experiences and participate in discussions with NVIDIA and other experts in the field.
Geforce Download For Mac
Online Forums